AI Automation: Bridging the Gap Between Promise and Practice
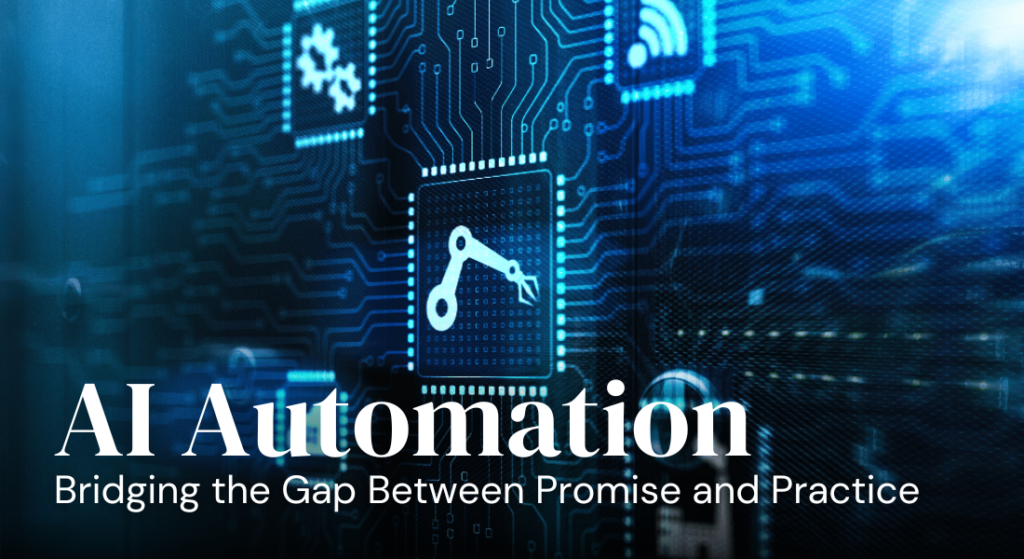
We’ve all probably seen countless posts on social media about how AI is coming to take our jobs. The internet was recently in a frenzy at how Devin is going to render software engineers useless in 6 months. The possibilities of a hyper intelligent AI running on a robot platform has had the imagination of Facebook pundits going wild for a while. But what is the actual state of AI in automation today and what does the road forward look like?
ChatGPT and its consequences: Humanity will never be the same
AI and machine learning has been around for ages. When a small AI startup in 2017 built a bot that could play Dota2 and beat some of the pros at their own game, no one really paid much attention except for a small community of gamers. However, this, amongst other small advancements in technology, led to a breaking point in the AI technology in 2022 when ChatGPT was released by that same small AI startup.
ChatGPT brought the concept of an LLM (or a large language model) to the forefront of the public consciousness and we’re still reeling from the consequences of that one move almost 2 years later. Since the release of ChatGPT everyone has heard of AI and tried to shoehorn it into every possible avenue of life and business. This has inevitably led to AI powered toasters and a website claiming to make millions selling pet supplies – no, wait, that was last time.
Revolution in Automation
There’s no denying that AI is leading to a revolution in the way that engineers think about automation. Where previously we would have had to rely on observational analysis to determine all possible situations that an automaton may have to face and limit those as much as possible using external factors; we can now simply train a model on the gathered observations and let the black-box do the hard part of determining outcomes.
AI allows for in-context handling of presented situations. For example, assume you have an automaton that gathers data from an RSS feed and summarizes it into a database for future reference. Previously, this automaton would have had to be coded to handle all possible data coming out of the RSS feed (for example, images, different text elements – paragraphs, headings etc.) With an LLM based approach, you’re able to pass all the data received from the RSS feed to the OpenAI API and generate a structured data output using the function calling approach and not have to worry about handling edge cases.
Broken Promises: The vision of utopia
While Sam Altman prepares for AGI, the truth is that right now, the applications for AI in most fields are very limited and don’t correspond to the lofty promises made in 2022. Most systems being implemented right now using AI are experimental and surface level. Even in these surface level implementation cases, we’ve seen some spectacular failures; For example, the recent cases of Air Canada and Chevy dealers using AI chat bots, in which hallucinations experienced by the AI models caused chatbots to behave in ways they were not originally designed for.
At the recent PMI Global Summit in Georgia, AI was a pervasive topic and PMI themselves launched Project Infinity which is a chatbot that allows you to poll responses from an existing database of project management concepts. According to Mike Griffiths, a prominent project management voice, there are 3 levels of AI automation:
- AI Assistants: Chatbots such as ChatGPT as well as custom trained LLMs such as the ill-fated Air Canada support bot. These are used to generate summaries and are generally useful for data retrieval, aggregation and lookup
- AI Augmentation: Where these same chatbots are implemented into a select few software and are given the agency to be able to make changes the software by interacting with its API based on user inputs. This is being implemented to some extent by most SaaS companies and software vendors, for example, Microsoft integrating co-pilot into all of their office products.
- AI Automation: Where an AI system is able to automate tasks based on provided stimuli without the need for human intervention / input.
According to Mike Griffith, he’s yet to see any useful examples of AI automation in the project management space and his observations are shared by similar keen observers in other industries: There is no actual, useful implementation of AI automation in the wild right now.
The future: “General Automation Intelligence”
As OpenAI and their competitors’ hurdle towards AGI at breakneck speed, it is important to remember that technological improvement for the sake of technological improvement leads to only failure and ruin in the short term. As alluded to before, the current AI landscape has striking resemblance to the Dotcom bubble of old. Every day new ‘innovative’ AI based solutions are released with very little actual real-world use cases for them. We seem to be at the peak of the Gartner Hype Cycle for AI.
This doesn’t necessarily mean that the technology being produced isn’t useful, just that the real-world implementation of the technology is years, perhaps decades, behind what the latest ventures in the AI space are doing. After all, only a decade and a half after the dotcom bust, the world had embraced the internet and trillions of dollars of wealth had been created.
The future, then, is in AI based automation. We’ve coined a term “General Automation Intelligence” GAI to refer to a hypothetical future technology which is one step beyond simple AI automation. While an AI based automation solution would have to be developed, tested and the constraints of its usage defined, a theoretical General Automation Intelligence would be able to understand automation requirements based on human input, determine the infrastructure needed, and solve any problems it faces. In this way, a GAI would be the theoretical real-world implementation of a theoretical artificial general intelligence – the theoretical muscle for the theoretical brain.
Conclusion
While the rise of AI has sparked imaginations and fueled aspirations of a future where tasks are seamlessly managed by intelligent systems, the reality remains tethered to the present. Despite notable advancements, the gap between promise and practice looms large. Broken promises and experimental applications serve as stark reminders that the road to true AI automation is riddled with challenges.
True progress requires more than just technological innovation; it demands careful consideration of ethical implications, responsible development practices, and thoughtful integration into society.
Syncropix is bringing state of the art technology related to Marketing, Management and Automation to the Pakistani market. We specialize in business process automation, management solutions and Artificial Intelligence solutions for small and large businesses alike.